What is AI? A Comprehensive Guide to Artificial Intelligence
Artificial Intelligence (AI) refers to the development of computer systems that can perform tasks that typically require human intelligence, such as visual perception, speech recognition, and decision-making.

The concept of AI has been around for decades, but recent advancements in machine learning and deep learning have led to significant breakthroughs in the field, making it a crucial part of modern technology.
As we explore the world of artificial intelligence, it's essential to understand its definition, history, and applications. This guide will provide a comprehensive overview of AI, delving into its various aspects and shedding light on its significance in today's world.
Understanding the Fundamentals of AI
Artificial Intelligence (AI) is revolutionizing the way we live and work, but to truly appreciate its impact, we must first understand its fundamentals.
The study of AI involves understanding its core definition and the principles that drive its development. At its core, AI is about creating systems that can perform tasks that typically require human intelligence.
The Core Definition of Artificial Intelligence
Artificial Intelligence refers to the development of computer systems that can perform tasks that would typically require human intelligence, such as visual perception, speech recognition, and decision-making.
Simulating Human Intelligence in Machines
The primary goal of AI is to simulate human intelligence in machines, enabling them to think and act like humans. This involves creating algorithms and statistical models that allow machines to learn from data, reason, and make decisions.
"The question of whether a computer can think is like the question of whether a submarine can swim." - Edsger W. Dijkstra. This quote highlights the complexity of defining intelligence in machines.
The Goal of Creating Smart Systems
The ultimate goal of AI is to create smart systems that can operate autonomously, making decisions based on data and improving their performance over time.
Intelligence vs. Artificial Intelligence
While intelligence refers to the natural ability of humans to learn, reason, and adapt, Artificial Intelligence is about replicating these abilities in machines.
Comparing Natural and Synthetic Intelligence
Natural intelligence is inherent in humans and some animals, allowing them to perceive, process information, and respond to their environment. Synthetic intelligence, on the other hand, is created by humans through complex algorithms and data processing.
The key differences between natural and synthetic intelligence lie in their origins, capabilities, and limitations. Understanding these differences is crucial for developing more sophisticated AI systems.
https://www.youtube.com/watch?v=qYNweeDHiyU
By grasping the fundamentals of AI, including its definition, goals, and the differences between natural and synthetic intelligence, we can better appreciate the potential and limitations of this technology.
What is AI and Explain in Detail?
Understanding AI requires delving into the science behind its systems, including computational models and algorithms. Artificial Intelligence is a multifaceted field that involves creating machines capable of performing tasks that typically require human intelligence, such as learning, problem-solving, and decision-making.
The Science Behind AI Systems
The science behind AI systems is rooted in complex computational models and algorithms that enable machines to process and analyze vast amounts of data. These models are designed to recognize patterns, make predictions, and improve their performance over time through various learning processes.
Computational Models and Algorithms
Computational models in AI are often inspired by the human brain's structure and function. Algorithms, such as neural networks and deep learning models, are crucial for processing complex data sets and achieving sophisticated AI capabilities.
Some of the key algorithms used in AI include:
- Decision Trees
- Random Forests
- Support Vector Machines (SVM)
- Neural Networks
Data Processing and Pattern Recognition
Data processing is a critical component of AI systems, as it involves cleaning, transforming, and analyzing data to extract meaningful insights. Pattern recognition is a key aspect of AI, enabling machines to identify trends, make predictions, and classify data into relevant categories.
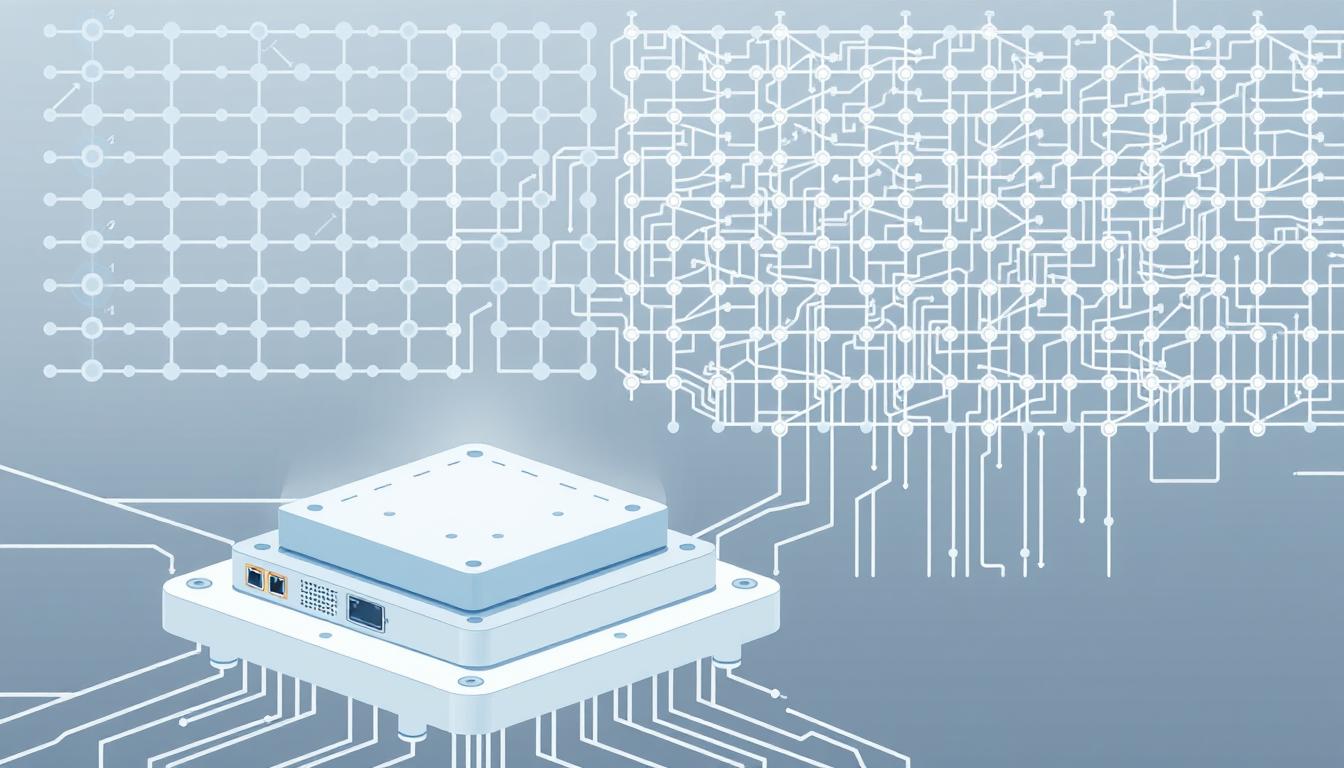
Key Components That Make AI Work
For AI systems to function effectively, they require both robust hardware and sophisticated software frameworks.
Hardware Requirements
The hardware requirements for AI systems are significant, often necessitating powerful processors, large memory capacities, and specialized hardware such as Graphics Processing Units (GPUs) and Tensor Processing Units (TPUs).
Software Frameworks and Tools
Software frameworks like TensorFlow, PyTorch, and Keras provide the necessary tools for developing, training, and deploying AI models. These frameworks offer a range of functionalities, from basic neural network layers to complex optimization algorithms.
Framework | Description | Key Features |
---|---|---|
TensorFlow | An open-source software library for numerical computation | Distributed training, automatic differentiation |
PyTorch | A dynamic computation graph and automatic differentiation system | Rapid prototyping, dynamic computation graph |
Keras | A high-level neural networks API | Easy to use, modular, extensible |
"The development of AI is a complex task that requires a multidisciplinary approach, combining insights from computer science, mathematics, and domain-specific knowledge."
The Historical Evolution of Artificial Intelligence
Tracing the evolution of AI reveals a fascinating journey from conceptual beginnings to the sophisticated technologies we see today. The history of artificial intelligence is marked by significant milestones, from early theoretical foundations to the current era of practical applications.
Early Concepts and Founding Fathers
The concept of AI has its roots in ancient myths and legends, but the modern study of AI began taking shape in the mid-20th century. Pioneers like Alan Turing and John McCarthy laid the groundwork for AI research. Turing's 1950 paper, "Computing Machinery and Intelligence," proposed the Turing Test, a measure of a machine's ability to exhibit intelligent behavior equivalent to, or indistinguishable from, that of a human.
The Dartmouth Conference of 1956
The Dartmouth Conference is often cited as the birthplace of AI as a field of research. Organized by John McCarthy, Marvin Minsky, Nathaniel Rochester, and Claude Shannon, this conference brought together leading researchers to explore the possibilities of creating machines that could simulate human intelligence.
AI Winters and Resurgences
Despite initial optimism, AI research faced significant challenges and funding cuts, leading to periods known as "AI Winters." These downturns were caused by the inability of early AI systems to meet the high expectations set by their proponents, coupled with limitations in computing power and data availability.
Funding Cycles and Research Challenges
AI research has historically been subject to cycles of funding and neglect. The field has faced numerous challenges, from the difficulty of replicating human cognition to the limitations of early computing hardware. However, each resurgence has brought new insights and advancements.
The Modern AI Revolution
The current AI revolution is driven by breakthroughs in big data and computing power. Modern AI systems leverage vast datasets and powerful processors to learn and improve, enabling applications that were previously unimaginable.
Big Data and Computing Power Breakthroughs
The availability of large datasets and advancements in computing hardware, particularly graphics processing units (GPUs), have been crucial to the success of modern AI. These developments have enabled the training of complex models that can perform tasks such as image recognition, natural language processing, and predictive analytics.
The evolution of AI is summarized in the following table:
Era | Key Developments | Impact |
---|---|---|
Early Concepts | Theoretical foundations, Turing Test | Established AI as a field of research |
AI Winters | Funding cuts, limited computing power | Slowed progress, shifted focus |
Modern AI | Big data, GPU advancements | Enabled sophisticated AI applications |
https://www.youtube.com/watch?v=Z9HKO7YoWCU
The historical evolution of AI is a testament to human ingenuity and the relentless pursuit of innovation. As AI continues to advance, understanding its history provides valuable context for appreciating current achievements and anticipating future developments.
Types of Artificial Intelligence Systems
From narrow AI to the hypothetical superintelligence, the types of AI systems vary significantly in their complexity and potential applications. Understanding these different categories is essential for grasping the current capabilities and future directions of AI.
Narrow AI vs. General AI vs. Superintelligence
Narrow AI, also known as weak AI, is designed to perform a specific task. It is the most common form of AI today, powering applications such as virtual assistants, image recognition systems, and language translation software.
Narrow AI operates within predetermined parameters and is not capable of general reasoning or decision-making beyond its programming. On the other hand, General AI, or strong AI, refers to a hypothetical AI system that possesses the ability to understand, learn, and apply knowledge across a wide range of tasks, similar to human intelligence.
Current Capabilities and Future Possibilities
Currently, narrow AI is prevalent, with applications in various industries. General AI, however, remains a subject of ongoing research and development. The potential for general AI to revolutionize numerous aspects of life is vast, but it also raises significant ethical and societal questions.
Type of AI | Description | Current Status |
---|---|---|
Narrow AI | Designed for specific tasks | Widely used in applications |
General AI | Hypothetical AI with human-like intelligence | Under research and development |
Superintelligence | Significantly surpasses human intelligence | Theoretical concept |
Reactive Machines and Limited Memory Systems
Reactive machines are a type of AI that react to the current state of the environment without forming memories or using past experiences to influence decisions. An example of a reactive machine is a chess computer that makes moves based on the current position of the pieces on the board.
"The development of AI is a double-edged sword, bringing about both immense benefits and significant challenges." - Expert in AI Ethics
How Chess Computers and Self-Driving Cars Work
Chess computers and self-driving cars are examples of AI systems that utilize different types of intelligence. Chess computers are reactive machines, while self-driving cars use limited memory systems to make decisions based on recent data, such as the position of other cars and road conditions.
Theory of Mind and Self-Aware AI
Theory of mind AI refers to systems that can understand and interpret the mental states of humans, such as beliefs, desires, and intentions. Self-aware AI takes this a step further by having a consciousness of its own existence and emotions.
The Frontier of AI Development
The development of theory of mind and self-aware AI represents the frontier of AI research. These advanced AI systems have the potential to significantly impact various aspects of society, from healthcare to education.
As AI continues to evolve, understanding the different types of AI systems is crucial for harnessing their potential while addressing the challenges they present.
Core Technologies Powering Modern AI
The backbone of modern artificial intelligence is formed by several key technologies that enable machines to learn, understand, and interact with the world. These technologies have revolutionized the field of AI, making it possible for systems to perform complex tasks that were previously the exclusive domain of humans.
Machine Learning Fundamentals
Machine learning is a crucial subset of AI that involves training algorithms to learn from data and make predictions or decisions without being explicitly programmed. This is achieved through various techniques, including supervised, unsupervised, and reinforcement learning.
Algorithms That Learn From Experience
At the heart of machine learning are algorithms that can learn from experience, improving their performance over time as they are exposed to more data. A key advantage of these algorithms is their ability to adapt to new information, making them highly versatile in a wide range of applications. For instance, in recommendation systems, these algorithms can learn user preferences over time, providing more personalized suggestions.
"Machine learning is a key driver of the AI revolution, enabling systems to learn from data and improve their performance over time."
Deep Learning and Neural Networks
Deep learning is a specialized form of machine learning that uses neural networks to analyze data. These networks are modeled after the human brain, with layers of interconnected nodes (neurons) that process and transmit information.
Mimicking Brain Structure for Complex Tasks
By mimicking the structure and function of the human brain, deep learning models can tackle complex tasks such as image recognition, speech recognition, and natural language processing.
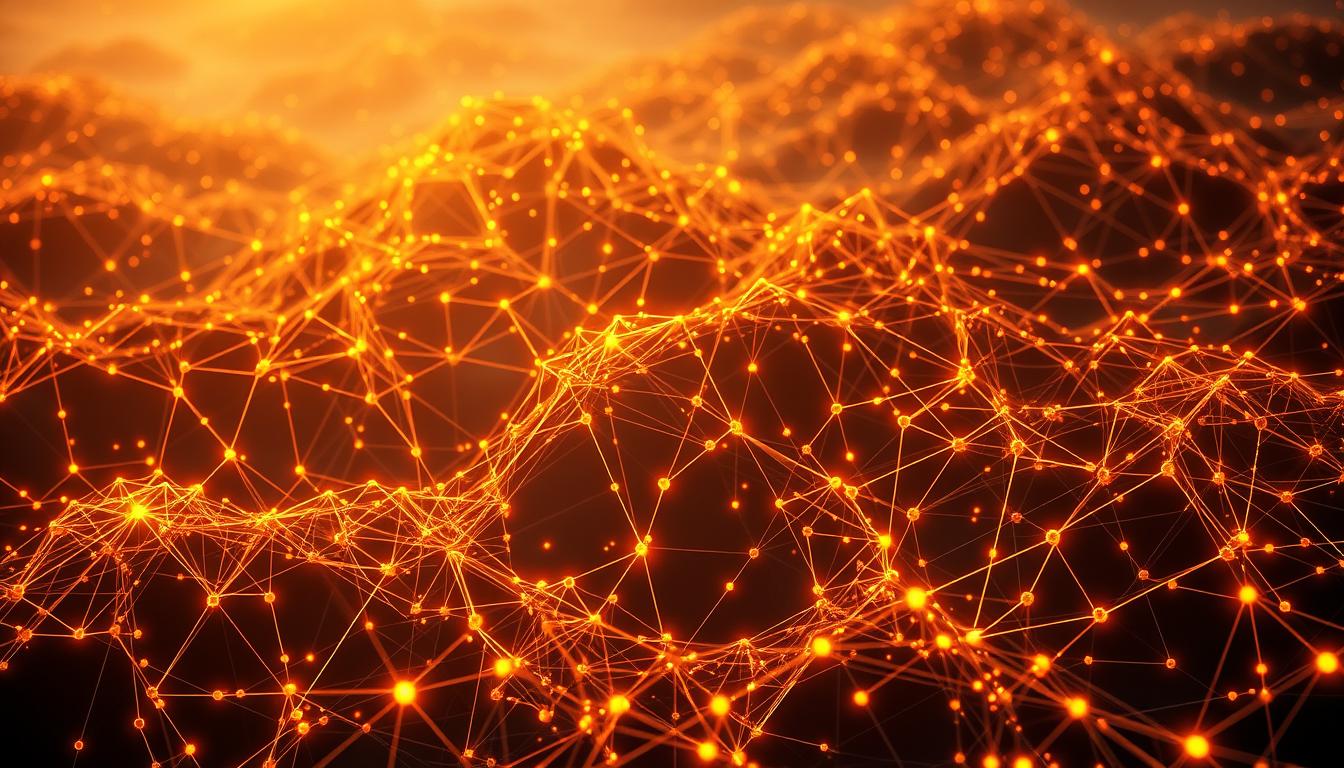
Natural Language Processing
Natural Language Processing (NLP) is a technology that enables computers to understand, interpret, and generate human language. This involves a range of tasks, from simple text processing to complex dialogue generation.
How Computers Understand Human Language
NLP combines machine learning and linguistic analysis to enable computers to comprehend the nuances of human language, including syntax, semantics, and context. This capability is crucial for applications such as chatbots, language translation, and text summarization.
Computer Vision and Image Recognition
Computer vision is another critical technology in modern AI, allowing machines to interpret and understand visual information from the world. This is achieved through image recognition algorithms that can identify objects, people, and patterns within images.
Teaching Machines to See and Interpret
By training on large datasets of images, computer vision systems can learn to recognize and classify visual content with high accuracy. This technology has numerous applications, including surveillance, healthcare, and autonomous vehicles.
How AI Algorithms Make Decisions
Understanding how AI algorithms make decisions is crucial for appreciating the capabilities and limitations of artificial intelligence.
Training Data and Learning Processes
The foundation of an AI algorithm's decision-making capability lies in its training data and the learning processes it employs. High-quality training data is essential for developing accurate and reliable AI models.
The Importance of Quality Data
The quality of the training data directly impacts the performance of an AI algorithm. Data that is diverse, accurate, and relevant enables the algorithm to learn effectively and make informed decisions.
Supervised vs. Unsupervised Learning
AI algorithms utilize different learning approaches, primarily supervised and unsupervised learning, to analyze data and make decisions.
Different Approaches to Machine Education
Supervised learning involves training the algorithm on labeled data, whereas unsupervised learning relies on the algorithm to identify patterns in unlabeled data. Each approach has its advantages and is suited to different applications.
Learning Approach | Description | Application |
---|---|---|
Supervised Learning | Trained on labeled data | Image classification, speech recognition |
Unsupervised Learning | Identifies patterns in unlabeled data | Clustering, anomaly detection |
Reinforcement Learning Models
Reinforcement learning represents another critical approach, where AI algorithms learn through trial and error by interacting with an environment.
Learning Through Trial and Error
This method allows the algorithm to adapt and improve its decision-making capabilities based on feedback received from its actions.
https://www.youtube.com/watch?v=o7ONJ5R6csc
Real-World Applications of AI Technology
Real-world applications of AI are revolutionizing industries, transforming the way businesses operate, and improving lives. From healthcare to finance, transportation, and retail, AI is being harnessed to drive innovation and efficiency.
AI in Healthcare and Medicine
AI is making significant inroads in healthcare, improving diagnosis accuracy, streamlining clinical workflows, and personalizing patient care. Machine learning algorithms can analyze vast amounts of medical data to identify patterns and predict patient outcomes.
Diagnosis, Drug Discovery, and Patient Care
AI-powered diagnostic tools are helping doctors detect diseases earlier and more accurately. In drug discovery, AI is accelerating the identification of potential new treatments by analyzing complex biological data. Additionally, AI-driven chatbots are enhancing patient care by providing personalized support and guidance.
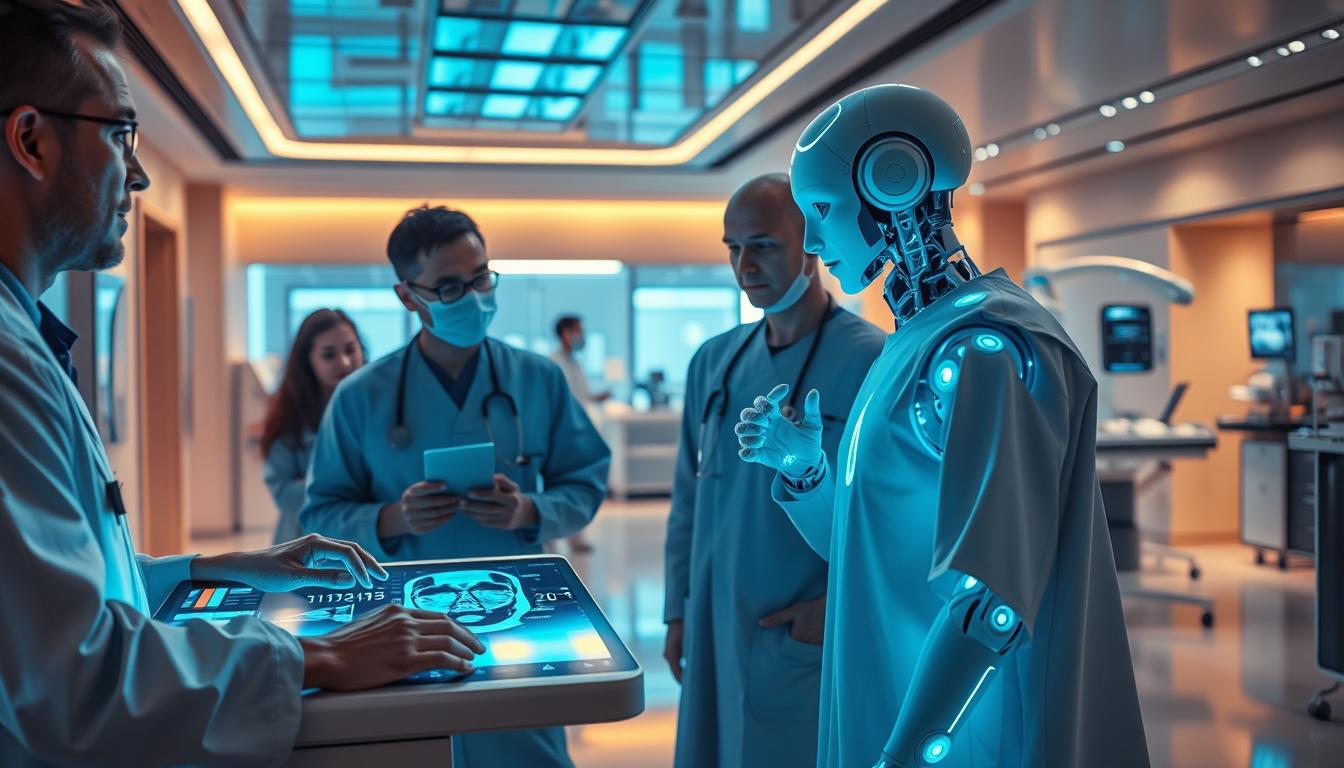
Financial Services and Banking Applications
The financial sector is leveraging AI to enhance security, improve customer experience, and optimize operations. AI-driven fraud detection systems can identify suspicious transactions in real-time, reducing the risk of financial crimes.
Fraud Detection and Algorithmic Trading
AI algorithms are being used to detect and prevent fraudulent activities, protecting both financial institutions and their customers. In algorithmic trading, AI systems analyze market data to make rapid, data-driven trading decisions.
Transportation and Autonomous Vehicles
The transportation industry is on the cusp of a revolution, driven by the development of autonomous vehicles. AI is being used to improve safety, reduce traffic congestion, and enhance the overall driving experience.
The Road to Self-Driving Cars
Self-driving cars rely on AI to interpret sensory data, navigate roads, and avoid obstacles. As the technology continues to evolve, we can expect to see significant advancements in the safety and efficiency of transportation systems.
Retail and Customer Experience
AI is transforming the retail industry by enabling personalized customer experiences, improving supply chain management, and optimizing inventory levels. AI-powered recommendation systems suggest products to customers based on their preferences and shopping history.
Personalization and Inventory Management
Retailers are using AI to tailor their marketing efforts and improve customer engagement. AI also helps in managing inventory by predicting demand and optimizing stock levels, thereby reducing waste and improving efficiency.
AI in Everyday Life: Tools You're Already Using
From virtual assistants to personalized recommendations, AI is revolutionizing the way we live and consume content. AI's integration into daily life is becoming increasingly seamless, making various tasks more efficient and personalized.
Virtual Assistants and Smart Home Devices
Virtual assistants like Siri, Alexa, and Google Assistant have become household names, making it easier to manage daily tasks, control smart home devices, and access information on the go.
Siri, Alexa, and Google Assistant
These virtual assistants use AI to understand voice commands, perform tasks, and provide relevant information. For instance, Siri can set reminders, send messages, and make calls, while Alexa can control lighting, temperature, and entertainment systems.
Recommendation Systems
Recommendation systems are another significant application of AI in everyday life, particularly in streaming and e-commerce platforms.
How Netflix and Amazon Predict Your Preferences
Netflix and Amazon use sophisticated AI algorithms to analyze user behavior and preferences, recommending content and products that are likely to interest them. This not only enhances user experience but also increases engagement and sales.
Platform | AI Application | Benefit |
---|---|---|
Virtual Assistants | Voice Recognition | Hands-free control, ease of use |
Netflix, Amazon | Recommendation Systems | Personalized content, increased engagement |
Social Media | Content Curation, Targeted Advertising | Relevant content, effective advertising |
Social Media Algorithms
Social media platforms use AI-driven algorithms to curate content and target advertisements based on user behavior and preferences.
Content Curation and Targeted Advertising
These algorithms analyze user interactions to display relevant content and ads, enhancing user experience and advertising effectiveness.
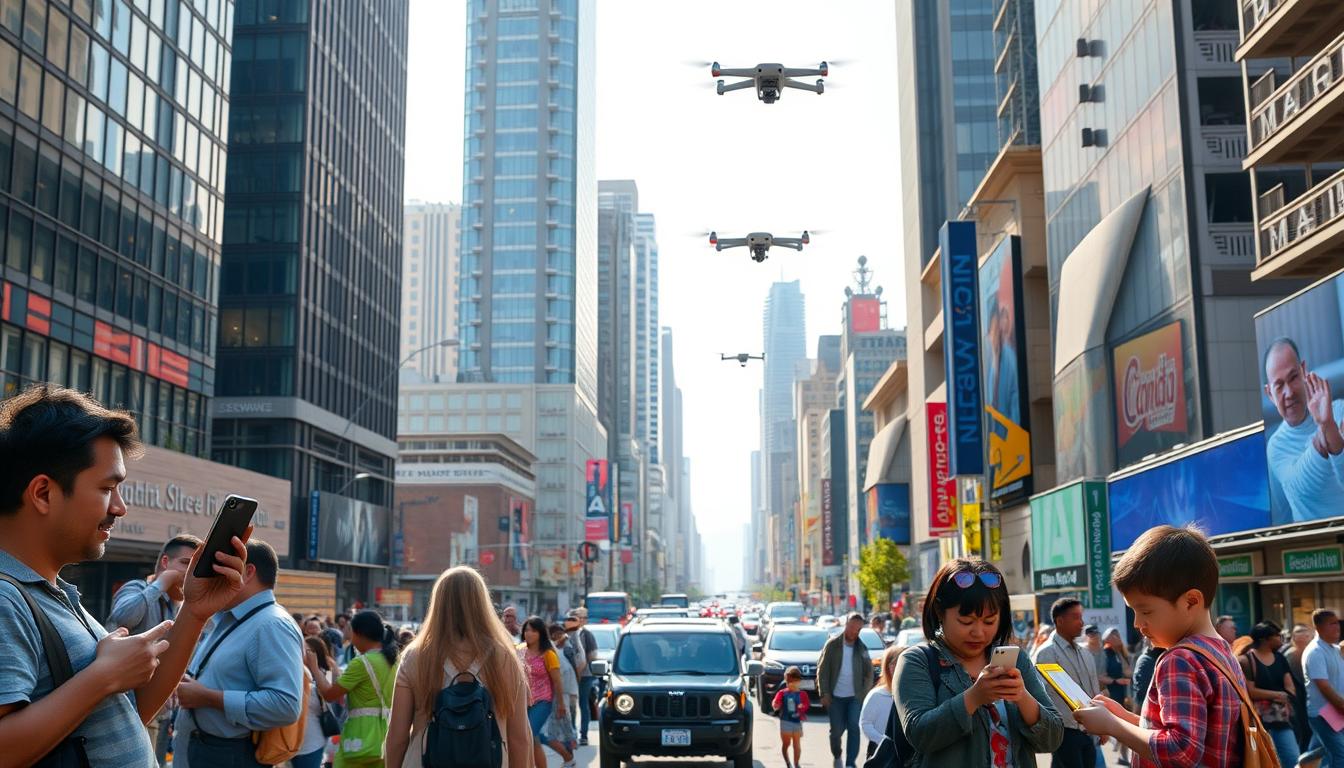
By understanding and leveraging these AI-driven tools, we can enhance our daily lives, making them more convenient, personalized, and enjoyable.
Ethical Considerations and Challenges
As AI continues to integrate into various aspects of our lives, it's crucial to address the ethical considerations and challenges that come with it. The development and deployment of AI systems raise important questions about their impact on society, privacy, and the economy.
The ethical landscape of AI is complex and multifaceted, involving issues that range from privacy concerns to bias in AI and job displacement. Addressing these challenges is essential to ensure that AI technologies are developed and used responsibly.
Privacy Concerns in the Age of AI
One of the significant ethical challenges associated with AI is the issue of privacy concerns. AI systems often rely on vast amounts of personal data to function effectively, raising concerns about data collection and surveillance.
Data Collection and Surveillance Issues
The collection and use of personal data by AI systems pose significant privacy risks. There is a need for transparent data practices and robust privacy protections to mitigate these risks.
- Implementing strong data protection regulations
- Ensuring transparency in data collection practices
- Providing users with control over their personal data
Bias and Fairness Issues
Another critical ethical issue in AI is the problem of bias in AI systems. Bias can lead to unfair outcomes and discrimination, undermining the potential benefits of AI.
Addressing Algorithmic Discrimination
To address algorithmic discrimination, it's essential to develop and implement fairness-aware AI systems. This involves identifying and mitigating bias in AI algorithms and ensuring that they are transparent and explainable.
Approach | Description | Benefits |
---|---|---|
Fairness-aware AI | Designing AI systems to identify and mitigate bias | Reduces discrimination and promotes fairness |
Transparent AI | Making AI decision-making processes understandable | Enhances trust and accountability |
Job Displacement and Economic Impact
The impact of AI on the workforce and economy is another significant ethical consideration. While AI has the potential to bring about numerous economic benefits, it also poses the risk of job displacement.
Preparing for Workforce Transformation
To mitigate the negative effects of job displacement, it's crucial to prepare the workforce for the changes brought about by AI. This involves investing in education and retraining programs that equip workers with the skills needed for an AI-driven economy.
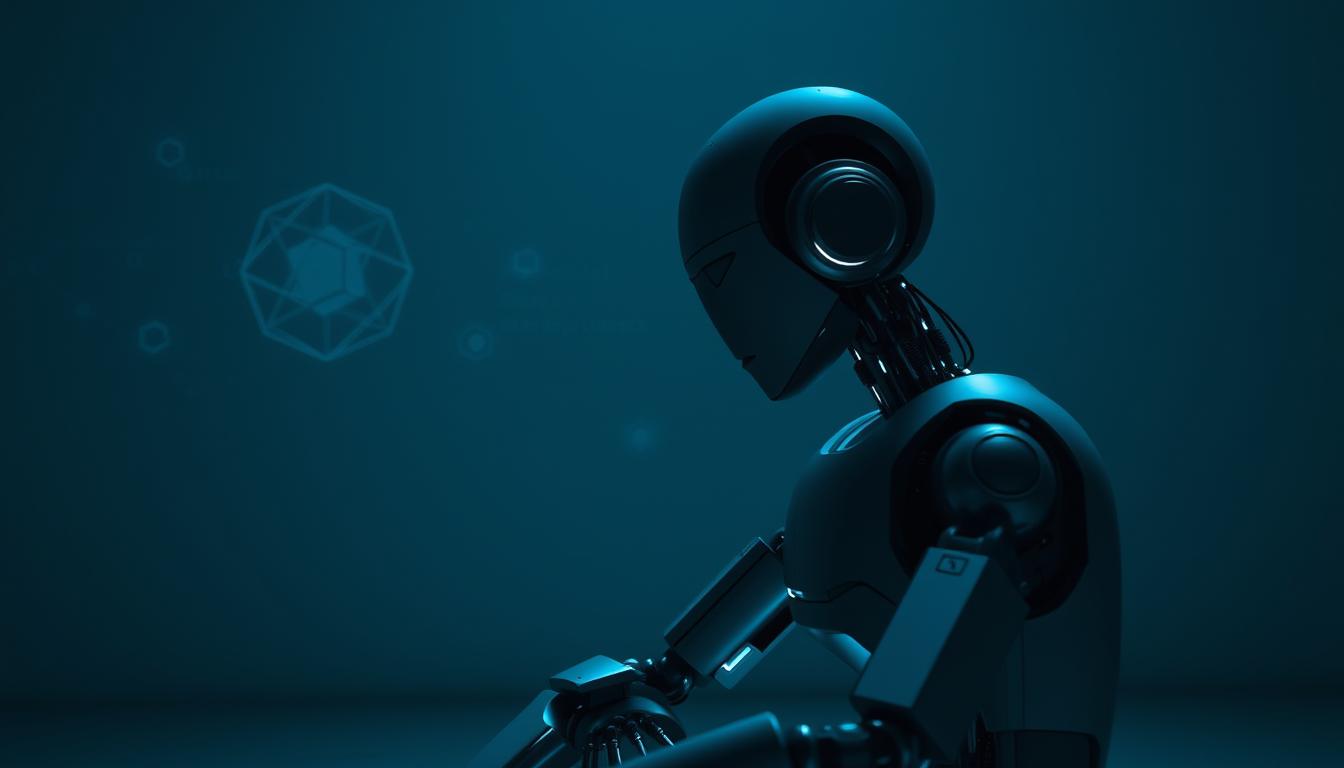
By addressing the ethical considerations and challenges associated with AI, we can ensure that these technologies are developed and used in ways that benefit society as a whole.
Getting Started with AI: Tools and Resources
The world of Artificial Intelligence is more accessible than ever, thanks to a variety of beginner-friendly platforms and resources. Whether you're a student, a professional looking to upskill, or simply an enthusiast, there's never been a better time to dive into AI.
Beginner-Friendly AI Platforms
For those new to AI, platforms like Google Colab, TensorFlow, and PyTorch offer an excellent starting point. These platforms provide not only the necessary tools but also extensive documentation and community support.
Google Colab, TensorFlow, and PyTorch
Google Colab is a free platform that allows you to write and execute Python code in the cloud. It's particularly useful for data science and machine learning tasks, providing access to GPUs and TPUs.
TensorFlow is an open-source software library for numerical computation, particularly well-suited and fine-tuned for large-scale Machine Learning (ML) and Deep Learning (DL) tasks.
PyTorch is another popular open-source ML library that provides a dynamic computation graph and is known for its simplicity and flexibility.
Platform | Key Features | Use Cases |
---|---|---|
Google Colab | Free, cloud-based, GPU/TPU access | Data science, ML tasks, educational purposes |
TensorFlow | Open-source, large-scale ML/DL | Production environments, complex ML models |
PyTorch | Dynamic computation graph, simplicity | Research, rapid prototyping, DL tasks |
Learning Paths for Different Skill Levels
Learning AI is a journey that caters to various skill levels. From novice to expert, there are numerous resources available, including online courses, tutorials, and certification programs.
From Novice to AI Practitioner
For beginners, starting with the basics of programming and understanding the fundamentals of AI is crucial. As one progresses, diving into specialized areas like ML and DL becomes the next step.
Essential Programming Languages for AI
Programming languages play a vital role in AI development. Among these, Python and R are particularly noteworthy.
Python, R, and Specialized Libraries
Python is widely regarded as the language of choice for AI due to its simplicity and the extensive libraries available, such as NumPy, pandas, and scikit-learn.
R is another popular language, especially among statisticians and data analysts, offering comprehensive libraries for data visualization and statistical analysis.
Specialized libraries like TensorFlow and PyTorch further enhance the capabilities of these languages, making complex AI tasks more manageable.
The Future Landscape of Artificial Intelligence
The future of AI is poised to revolutionize numerous aspects of our lives. As we move forward, it's essential to understand the emerging trends and technologies that will shape the AI landscape.
Emerging Trends and Technologies
The field of AI is rapidly evolving, with several emerging trends and technologies gaining prominence. One such area is the integration of AI with quantum computing, which promises to solve complex problems that are currently unsolvable.
Quantum Computing and AI
Quantum computing has the potential to exponentially increase the processing power available to AI systems. This could lead to breakthroughs in areas like drug discovery, climate modeling, and optimization problems.
Potential Breakthroughs on the Horizon
Several potential breakthroughs are on the horizon, including advancements in neuromorphic computing. This type of computing is inspired by the structure and function of the human brain, potentially leading to more efficient and adaptive AI systems.
Neuromorphic Computing and Beyond
Neuromorphic computing could enable AI systems to learn and adapt in real-time, without the need for extensive retraining. This has significant implications for applications like robotics and autonomous vehicles.
AI's Role in Solving Global Challenges
AI is poised to play a critical role in addressing some of the world's most pressing challenges. From climate change to healthcare and education, AI can help analyze complex data, identify patterns, and develop effective solutions.
Climate Change, Healthcare, and Education
In climate change, AI can help model and predict the effects of different mitigation strategies. In healthcare, AI can assist in diagnosis and personalized medicine. In education, AI can enable personalized learning experiences, improving outcomes for students worldwide.
Debunking Common Myths About AI
The portrayal of Artificial Intelligence (AI) in media often blurs the line between reality and science fiction, leading to widespread misconceptions.
AI vs. Science Fiction Portrayals
Separating Hollywood from Reality
AI is often depicted in movies and TV shows as either a utopian solution to all human problems or a dystopian force that could destroy humanity. However, the reality of AI is more nuanced.
For instance, AI systems are not autonomous entities that can decide their own objectives or actions without human input. They are programmed to perform specific tasks based on the data they are trained on.
"The biggest risk with AI isn't that it will become self-aware and decide to destroy humanity, but rather that it will be used to exacerbate existing social issues if not developed responsibly."
Realistic Expectations for AI Development
The Pace and Direction of Progress
Understanding the current state of AI development is crucial for setting realistic expectations. Currently, AI is advancing rapidly in areas like machine learning and natural language processing.
The progress in AI is not linear; it is driven by breakthroughs in algorithms, increases in computational power, and the availability of large datasets.
Area | Current State | Future Potential |
---|---|---|
Machine Learning | Advanced pattern recognition and prediction capabilities. | Potential for more sophisticated decision-making processes. |
Natural Language Processing | Improved chatbots and virtual assistants. | More accurate and context-aware language understanding. |
Understanding AI Limitations
What AI Can and Cannot Do
While AI has made significant progress, it is not a panacea for all technological or societal challenges. AI systems are limited by their training data and the objectives they are designed to achieve.
By understanding these limitations and the current capabilities of AI, we can better navigate the complex landscape of AI development and application.
Conclusion
As we have explored throughout this comprehensive guide, Artificial Intelligence (AI) is transforming the world around us. From understanding the fundamentals of AI to examining its current applications and future potential, it's clear that AI is more than just a technological advancement - it's a catalyst for change across various industries.
The AI summary provided in this article highlights the significant progress made in areas such as machine learning, natural language processing, and computer vision. These technologies are not only enhancing existing processes but also enabling new possibilities in fields like healthcare, finance, and transportation.
Looking ahead to the future of AI, it's evident that we are on the cusp of a new era of innovation. As AI continues to evolve, we can expect to see even more sophisticated applications that will further integrate AI into our daily lives. Understanding AI's capabilities and limitations will be crucial for harnessing its potential and navigating the challenges it presents.
FAQ
What is Artificial Intelligence (AI)?
Artificial Intelligence refers to the development of computer systems that can perform tasks that typically require human intelligence, such as visual perception, speech recognition, decision-making, and language translation.
How does AI work?
AI systems work by using algorithms and data to make predictions, classify objects, and generate insights. They can be trained on large datasets to learn patterns and relationships, enabling them to make decisions or take actions.
What are the different types of AI?
There are several types of AI, including Narrow or Weak AI, General AI, and Superintelligence. Narrow AI is designed to perform a specific task, while General AI is a hypothetical AI system that can perform any intellectual task that a human can. Superintelligence is a hypothetical AI system that is significantly more intelligent than the best human minds.
What is Machine Learning?
Machine Learning is a subset of AI that involves training algorithms on data to enable machines to learn from experience and improve their performance on a task.
How is AI used in real-world applications?
AI is used in a wide range of applications, including healthcare, finance, transportation, and retail. Examples include diagnosis and treatment recommendations, fraud detection, self-driving cars, and personalized product recommendations.
What are the benefits of AI?
The benefits of AI include improved efficiency, enhanced decision-making, and increased accuracy. AI can also help to automate repetitive tasks, freeing up humans to focus on more complex and creative work.
What are the challenges and limitations of AI?
The challenges and limitations of AI include data quality issues, bias, and the need for transparency and explainability. AI systems can also be vulnerable to cyber attacks and data breaches.
How can I get started with AI?
To get started with AI, you can explore beginner-friendly platforms such as Google Colab, TensorFlow, and PyTorch. You can also take online courses or attend workshops to learn more about AI and its applications.
What is the future of AI?
The future of AI is likely to be shaped by emerging trends and technologies, including quantum computing, neuromorphic computing, and the increasing use of AI in industries such as healthcare and finance.
How will AI impact my job or career?
AI is likely to automate some tasks and change the nature of work in various industries. However, it is also likely to create new job opportunities and require workers to develop new skills.
What are the ethical considerations surrounding AI?
The ethical considerations surrounding AI include issues related to bias, fairness, transparency, and accountability. There are also concerns about job displacement, data privacy, and the potential for AI to be used in ways that are detrimental to society.